Topic
Office of the CFO
Automation
Published
November 2024
Reading time
18 minutes
AI Agents-Breaking Down the Verticals
Rewiring Organizations
Authors
Maximiliane Mainusch
Fellow
AI Agents-Breaking Down the Verticals
Download ArticleResearch
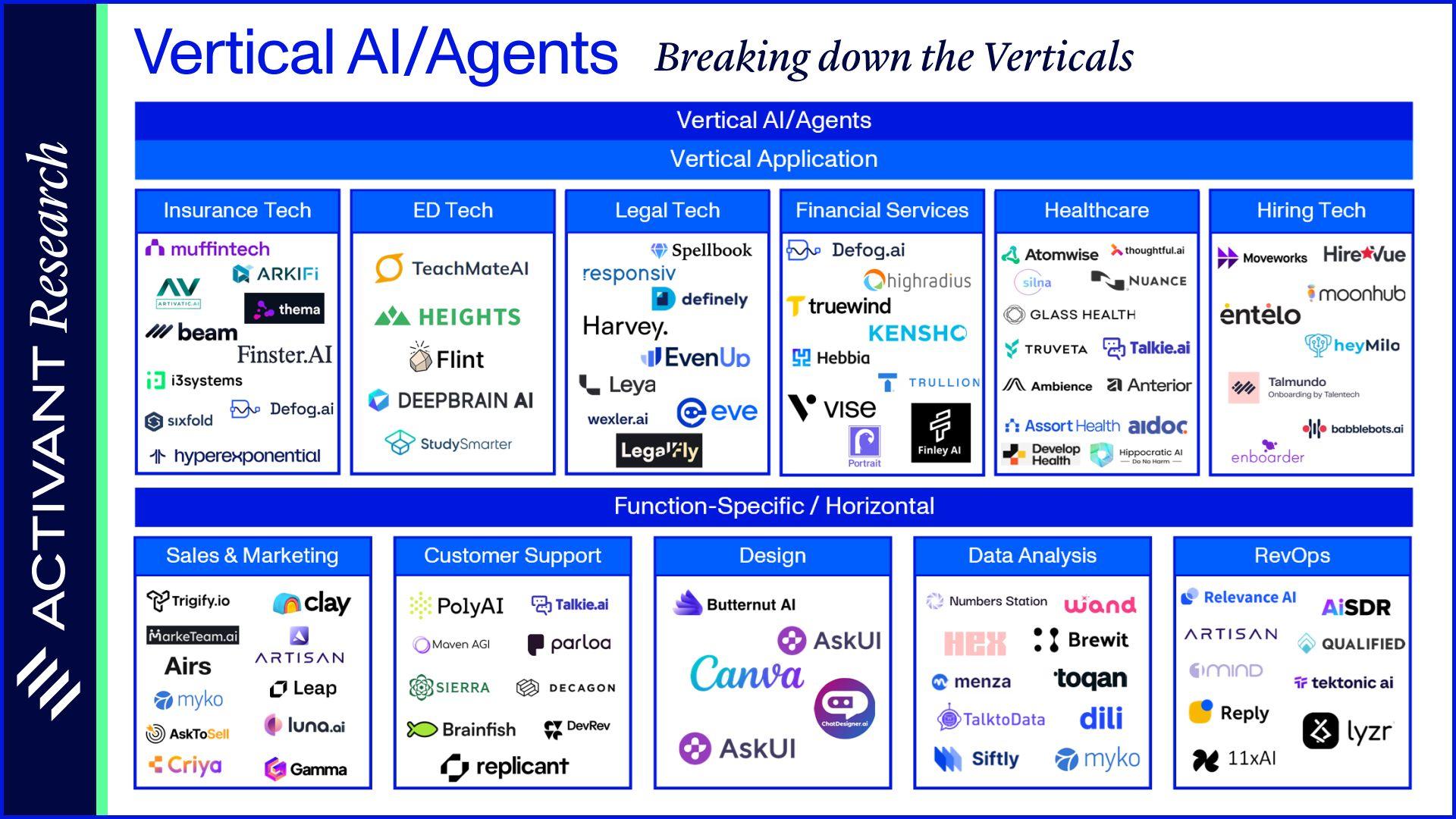
We often mistakenly believe that AI will simply automate some jobs and augment others. However, the introduction of AI agents reveals a far more nuanced and transformative potential. This isn't just another technological advancement—AI agents represent the next evolution of outsourcing, offshoring, contracting, and technology replacing people, all with a focus on vertical integration. This shift marks a significant change in how we think about workforce management and job functions in the age of AI.
Is "Vertical/Function Specific" the Buzzword for AI Agents?
Between 2000 and 2005, SaaS began to gain traction with companies like Salesforce pioneering cloud-based CRM, initially offering generic functionalities. By the late 2000s, as the cloud market matured, businesses recognized the need for industry-specific solutions, leading to the emergence of vertical SaaS. The early 2010s saw significant growth in vertical SaaS, and by the late 2010s, there was a notable expansion and integration of these solutions.
While it took nearly two decades for applications in software to shift from horizontal to vertical, the integration of AI has accelerated this transition. AI-driven vertical solutions have proliferated rapidly due to their ability to leverage industry-specific data. This has led to a surge in demand not just for vertical solutions, but also for company-specific applications.
Much like SaaS, which evolved from horizontal to vertical applications, AI agents are following a similar trajectory. Initially, AI tools like ChatGPT offered broad, horizontal applications. Now, we see a growing trend towards niche AI applications tailored to specific sectors.
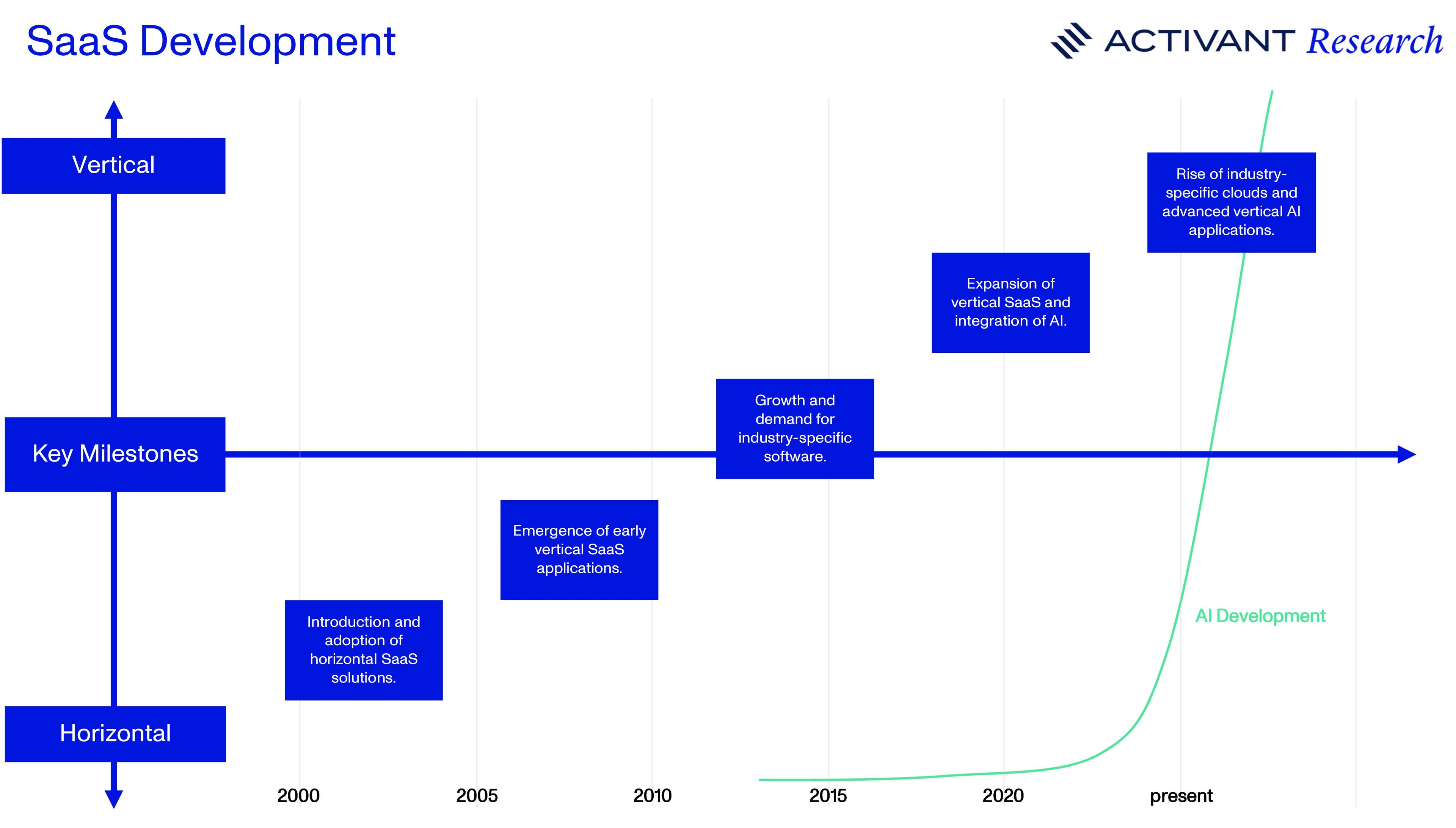
The evolution of the AI market since 2022 makes one thing clear: vertical AI agents are here to stay. However, the crucial question is whether legacy incumbents like Salesforce and Oracle can adapt quickly enough to meet the burgeoning demand for industry-specific AI applications. Or will startups, with their focus on single industries or functions, emerge as the true winners?
Agents Becoming Managers?
To understand the impact of AI agents on corporate structures, it's crucial to grasp the role of human managers and the limitations AI previously faced. Managers have two core job functions:
- Coordination challenge: Coordinating activities across various isolated workflows, integrating siloed data.
- Goal-seeking challenge: Using these integrated workflows to make decisions and take actions toward achieving objectives.
In essence, managers excel at re-bundling workflows to drive business goals. Previously, AI could only assist with the first function, coordinating and providing insights from siloed data, as it lacked the ability to seek and achieve goals. AI agents have now overcome this limitation, enabling them to perform core managerial functions effectively.
The challenge with vertical AI agents is that not all verticals are equally suited for AI-driven opportunities. Effective management relies on two key factors:
- Managerial capabilities: The skills and abilities required to complete tasks.
- Resource accessibility: Easy access to all necessary resources and siloed data.
For vertical AI to effectively serve a specific role akin to a human manager, it depends on:
- Agent sophistication: The advanced capabilities of the AI agent.
- Interoperability: The level of integration and open access to third-party resources within the domain.
Many industries lack either broad interoperability, sophisticated AI agents, or both. Consequently, some sectors and domains naturally favor existing incumbents, particularly where SaaS providers have already established strong hub positions. This inherent advantage makes it more challenging for vertical AI agents to disrupt these areas successfully.
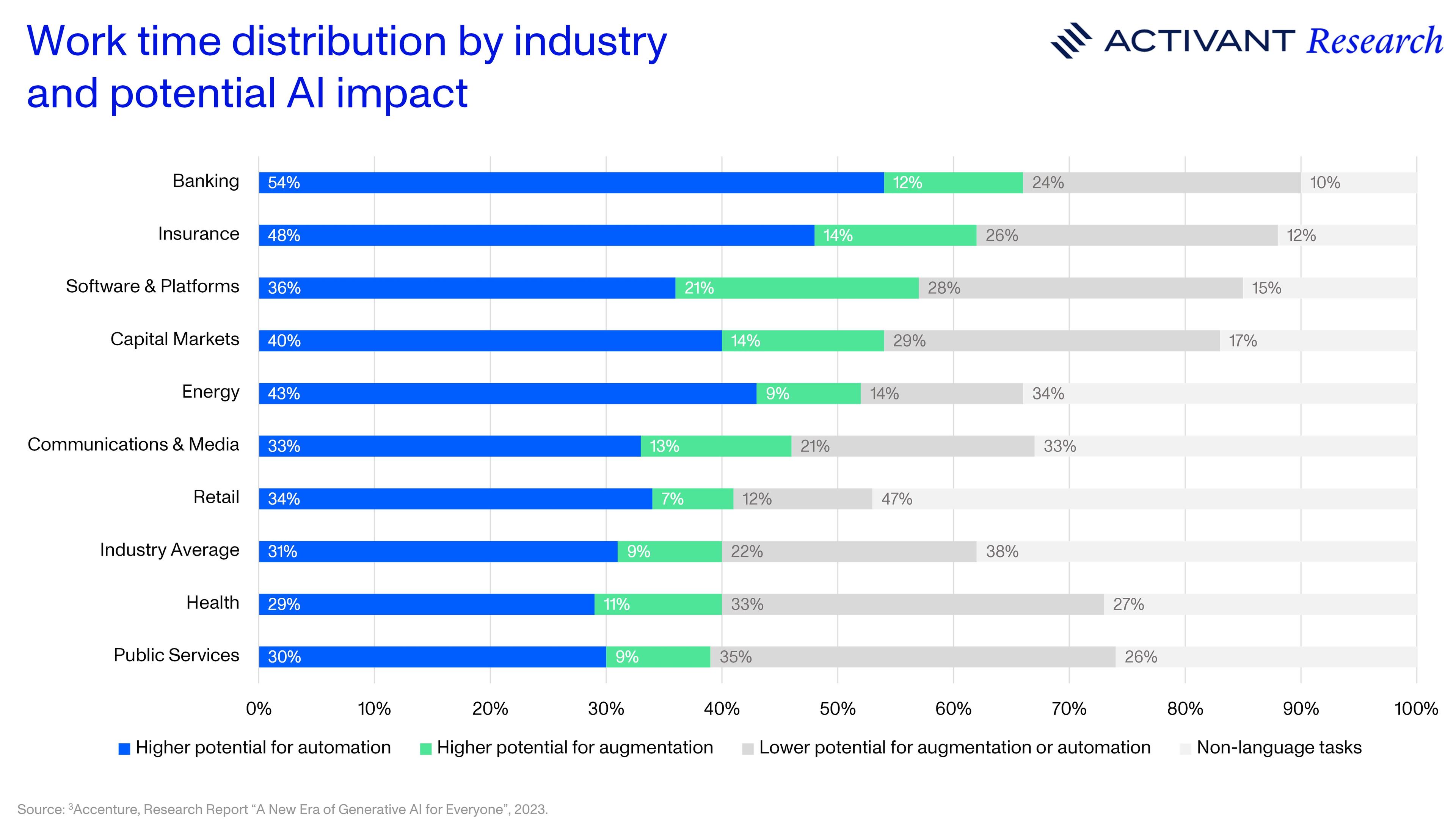
A 2023 study by Accenture on the transformative potential of generative AI in business identified the banking, insurance, software & platforms, and capital markets industries as having the highest potential for automation and augmentation.
Who is Going Vertical?
Beyond the financial and insurance sectors, significant developments are also emerging in the legal, edtech and hiring industries. Currently, the AI agent market landscape is dominated by players who either focus on specific verticals or excel in horizontal capabilities. While we have evaluated several pioneering players in these fields, we recognize that this market environment is very dynamic. New industries and companies are continually evolving, demonstrating advanced capabilities. Notably, there is strong movement in heavily regulated industries, where advancements in AI-driven efficiency were unforeseen just a few years ago.
Market Map
Vertical AI/Agents-Breaking Down the Verticals
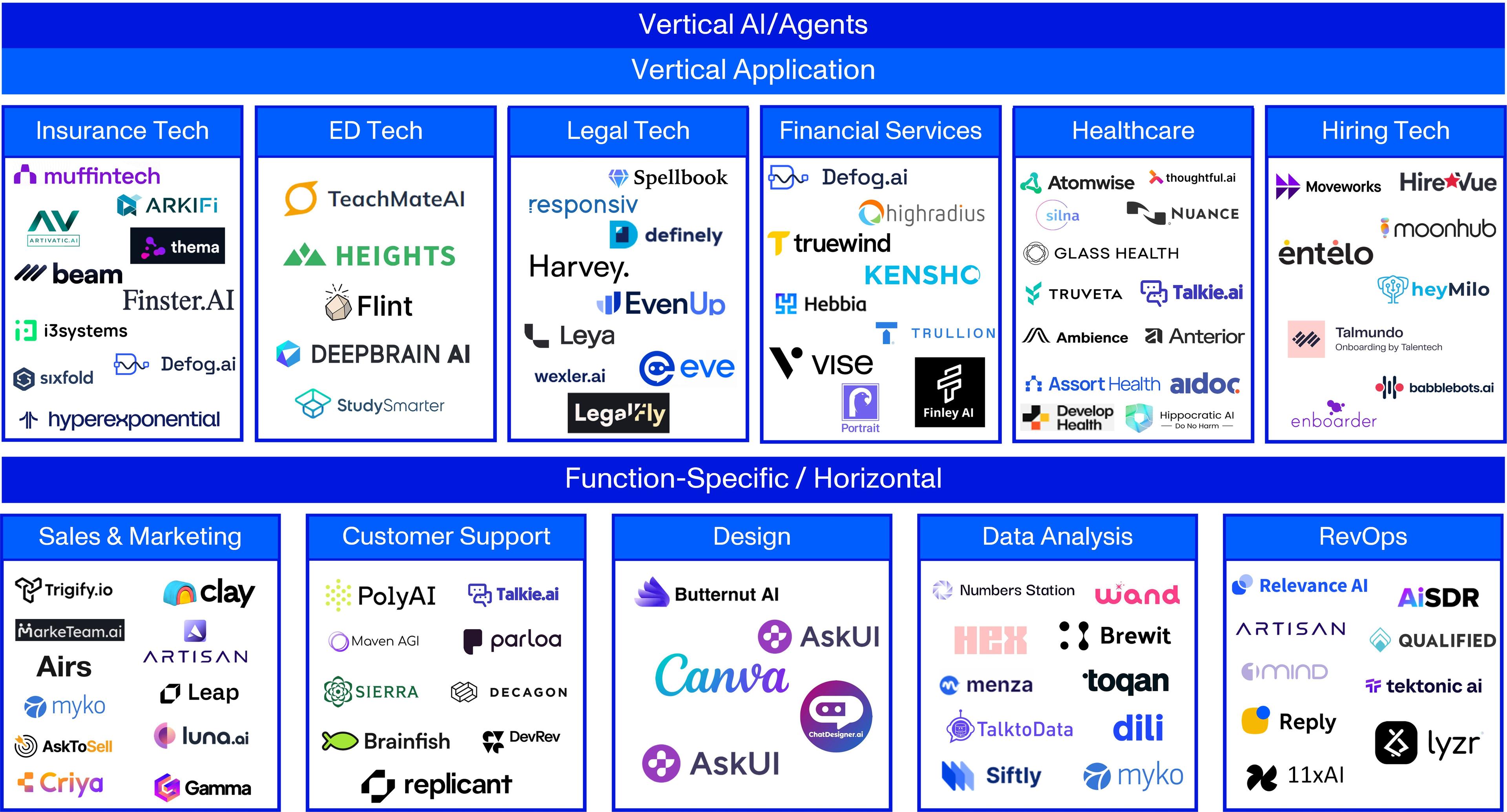
Legal
This sector has seen significant funding rounds and acquisitions, including Harvey’s $100m Series C, as well as early-stage investments in AI legal platforms such as Leya ($25m Series A), Legalfly ($16m Series A), and Definely ($9m Series A), as well as the $650m purchase of Casetext by Thomson Reuters. While industry experts believe there is huge potential for AI to provide legal advice, approximately 95% think that having AI make final decisions in court or in matters of legal, tax, fraud, risk, and compliance would be “a step too far”.1 Thus, the human-in-the-loop model remains indispensable.
Use Case 1) Replacing human legal assistants
Harvey offers a suite of AI products specifically tailored to lawyers and law firms, addressing all practice areas and workflows. Their custom-trained large language models (LLMs) execute legal tasks and track productivity based on industry-specific KPIs. The AI agent can draft texts, analyze documents, and answer questions in natural language, with a strong emphasis on correct citation and relevant sources. The success of Harvey's custom case law model highlights the significance of tailoring AI solutions to specific industries and use cases. The collaboration between Harvey, OpenAI, and the combined expertise of lawyers and AI researchers has produced a model that outperforms generic foundation models in terms of relevance, accuracy, and source citation. With their recent funding announcement, they plan to scale across other business functions.
Insurance
In the insurance industry, AI agents range from chatbots assisting with customer service to autonomous systems managing claims and policy underwriting. These agents leverage adaptive learning, decision-making, and end-to-end process automation, embodying the concept of agentic automation. It is a rather mature field, seeing that incumbents such as IBM and Salesforce are offering insurance-specific chatbots and agents that are pre-trained with industry specific data.
Use case 1) Offering industry-specific customer service
Muffintech is a conversational AI tool specifically designed for the insurance industry, primarily focusing on customer support, sales, and operations. Use cases include chatbots for customer interaction, lead generation, and internal knowledge bases. They are going a step further with their “Personal Autonomous User Self-Service” AI agent. This agent integrates with the CRM system, allowing it to make changes to a customer's insurance plan or profile upon request.
Use case 2) Automating claims processing
Some companies that offer multi-industry AI agents, such as Cognigy, also offering specialized AI agent solutions for the insurance industry. These solutions cover a range of service use cases, including claims processing and answering coverage questions. Cognigy also utilizes AI vision tools to detect license plates and analyze photos of damages for claims. Previously, claims processing involved manual tasks like analyzing unstructured data from various sources such as bills, invoices, and medical documents. AI now automates these repetitive tasks, extracting necessary data and categorizing it for efficient claims processing, significantly reducing the time and effort required from human agents.
Use Case 3) Replacing human insurance assistants
End-to-end solutions that combine previous efforts and technical capabilities in the sector are emerging, providing a solution that moves towards fully replacing agents in the insurance sector. Players like Beam offer a toolset of vertical-specific pretrained AI agents that can be integrated and connected to create workflows from claims management to AML/KYC automation.
Edtech
When it comes to the role of AI Agents in education, their primary function is to supplement the learning experience and extend the support available to teachers. These Agents can aid in content delivery, provide instant feedback, facilitate communication, and enable personalized learning pathways. While the initial data used in education can often be standardized, the value here lies in the personalization of each individual’s unique learning speed and need for further depth in specific areas. AI can be beneficial in pattern recognition and can push the limits when utilizing this information to create individualized recommendations. Especially with children, the personalized exchange with an AI assistant can create a human-like feeling, required for adaptive learning similar to engaging with a teacher. As technology continues to evolve, so will the capabilities of AI Agents. In this vertical these could be advancements in natural language processing, adaptive learning algorithms, and the integration of virtual and augmented reality.
It is notable that 2021 saw a historic peak in VC investment in Edtech, reaching $20.8 billion, driven by the demand for digital and automated learning tools during the Covid-19 pandemic. Since then, investments have significantly declined, which is a natural progression. This decline can be attributed to the previous surge of companies in this sector and an increased desire for more personal and hybrid interactions following the pandemic.2 It remains to be seen if new AI assistants can replicate this human-like interaction and revitalize the industry's investment potential.
Use Case 1) Enhancing operational efficiency
Players like Heights build a platform where one can monetize knowledge by creating educational content and building learning paths, enabled by AI. What is interesting in Heights’ product offering is the strong degree of automation that can be generated, eliminating annual tasks such as editing, community management and payment processing, so that educators can concentrate on their core skillset of creating educational content.
Use Case 2) Creating educational video content
Other players, such as DeepBrain AI, utilize AI in the field of content creation, reducing manual work by using AI Avatars to display content. The software is targeted at educators, assisting them increasing tutoring videos to build indefinitely accessible and scalable education channels. Going beyond simply creating the videos with text-to-speech tools, the avatars can answer questions and hold conversations on the education topics. The AI tutor can either be connected to an existing chatbot or a user can create a custom LLM based on the educational materials. Video templates showcase a first step into other functional sectors such as social media or marketing.
Use Case 3) Personalizing learning experiences (replacing teachers)
Taking one step further towards where we see the future of AI in Edtech leads to AI agents taking over the role of actual teachers or educators. This entails utilizing AI to optimize and individualize learning paths, combined with virtual teachers who communicate educational content. Flint, for instance, demonstrates such features. It is an AI tutoring platform built for schools, leveraging both existing and individual data to provide personalized education. By offering individual feedback and customized learning cycles, the software extends the capabilities of teachers, enabling them to cover more than they could alone. Flint also integrates various AI features, including text-to-speech, speech-to-text, language translation, and image generation with DALL-E 3.
Finance
AI implementation in the finance sector has evolved considerably from rule-based systems used for automating routine tasks. With the rise of machine learning and deep learning, AI has entered a transformative phase. Nowadays, AI agents are adept at analyzing large datasets, identifying patterns, and making real-time decisions. The financial sector holds immense potential for AI agents, addressing issues like productivity loss from frequent task switching and high automation potential. It is predicted to be the industry most significantly transformed by generative AI in the coming years, with the highest potential for AI-led job displacement.3
Use Case 1) Performing predictive analytics in finance and investment
Currently, we see most of AI being deployed in the finance sector in the field of predictive analytics. Solutions such as Defog and Kensho are used by banks and investment firms to analyze market trends, assess risks, and evaluate economic indicators, aiding in portfolio optimization and strategic decision-making. These AI solutions go beyond mere data analysis by offering recommendations and forecasting trends, leveraging machine learning for enhanced insights.
Use Case 2) Engaging with employees and customers
Players like Finley AI are adding the human touch by offering voice agents that act as knowledge providers. These agents deliver real-time market data and engage both internally and externally, offering financial guidance. With access to real-time stock data, market news, and insights, Finley AI serves as a productivity enhancement tool internally, providing financial data, detailed investment insights, and financial knowledge to support better decision-making. Externally, Finley AI functions as a customer service agent, keeping clients updated on financial data and offering personalized guidance on their financial decisions.
Healthcare
As one of the largest global industries, healthcare holds limitless potential for AI agents, especially as rising medical costs for government generates an inherent need for cost cutting. We have already started to see AI become integral across various sub-sectors, enhancing diagnostics in pathology, imaging, and radiology; enabling personalized medicine; accelerating drug discovery; providing virtual health assistance; and facilitating remote patient monitoring. Additionally, AI can streamline administrative tasks like medical documentation and billing and contributes to medical education and training. The entire healthcare value chain has numerous use cases for AI agents. With integration into healthcare IoT and support for ethical decision-making, AI has the potential to transform the entire sector.
Use Case 1) Automating inbound calls in clinics
AI agents are often associated with reducing administrative burdens through tasks like documentation, conversation intelligence, and appointment booking across sectors horizontally. Similarly, in healthcare, Assort Health, Hyro, JIQ, and Talkie.ai specifically automate inbound calls. Additionally, companies like Nuance use AI to transcribe and manage medical records.
Use Case 2) Analyzing medical images
AI's potential extends far beyond these applications. In imaging and radiology, Aidoc and Zebra Medical (acquired by Nanox) utilize AI to analyze medical images (X-rays, MRIs, CT scans). Paige has developed AI tools for pathology, aiding in the examination of tissue samples to identify cancer cells, with specific tools for prostate and breast cancer.
Use Case 3) Discovering and developing new drugs.
Personalized medicine is advancing with Atomwise, which uses AI to revolutionize drug discovery.
The healthcare industry is undergoing a transformative shift with voice AI technology, especially for patient access calls. Voice AI solutions, like those provided by Talkie, are making it possible for hospitals and clinics to offer 24/7 access to essential services, from appointment scheduling, prescription refills through to call navigation and medical triage. By automating routine calls, we not only address critical issues like staff shortages and long wait times but also enhance the patient experience, providing immediate support when they need it most. This level of access and efficiency is critical for modern healthcare, where timely, reliable communication is paramount.”
The healthcare industry is undergoing a transformative shift with voice AI technology, especially for patient access calls. Voice AI solutions, like those provided by Talkie, are making it possible for hospitals and clinics to offer 24/7 access to essential services, from appointment scheduling, prescription refills through to call navigation and medical triage. By automating routine calls, we not only address critical issues like staff shortages and long wait times but also enhance the patient experience, providing immediate support when they need it most. This level of access and efficiency is critical for modern healthcare, where timely, reliable communication is paramount.”
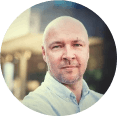
Pawel Lipinski, CEO, Talkie.ai
Hiring
One of the most developed functional use cases of AI agent deployment is in hiring. AI has been integrated into the hiring processes of major corporations like McKinsey, the Big Four accounting firms, and large U.S. investment banks for several years. Given the numerous repetitive tasks involved, hiring was one of the first business applications of AI. The primary goals are to streamline the hiring process, reduce biases, and provide data-driven insights for better hiring decisions. Despite a mature market, numerous startups are innovating within various hiring tasks or offering end-to-end solutions.
Use Case 1) Automating application processes
To reduce bias, Textio suggests unbiased language for job descriptions and communication, while BlendScore provides analytics to improve diversity in hiring. In candidate sourcing, AI agents scrape job boards, social media, and websites to identify potential candidates. Entelo finds and engages passive candidates through data analysis, and hireEZ aggregates candidate information from multiple platforms. For automated resume screening, HireVue ranks candidates.
Use Case 2) Automating interview assessment and candidate quality assurance
For interview scheduling. candidate assessment is enhanced by AI agents conducting initial evaluations through chatbots, video interviews, and skill-based tests, with HeyMilo applying various tools to assess candidates without HR presence. Pymetrics uses neuroscience-based games with AI to assess cognitive and emotional traits.
Use Case 3) Onboarding of new employees.
For employee onboarding, Talmundo and Enboarder use AI to provide new hires with personalized information, training, and compliance documentation.
Regulations Remain a Stumbling Block for AI Agents
Regulations remain a significant hurdle in the broad implementation of AI agents, as various industries face unique yet similar challenges. The finance and insurance sectors are particularly heavily regulated, especially banks and institutions, where managing sensitive data elevates the importance of data security. Similarly, the legal sector faces significant regulatory constraints, particularly regarding privacy and data protection due to the sensitive nature of legal data. Additional concerns include algorithmic transparency, cybersecurity vulnerabilities, and the potential for unfairness, bias, and discrimination.
While the educational sector emerges as one of the leading sectors in the adoption of AI and AI agents, it faces challenges primarily related to the loss of interpersonal connection and emotional factors. Furthermore, the disparity between the potential of Edtech and its reality is shaped by limited budgets and inadequate technical infrastructure, particularly in underprivileged geographics.
In general, the most substantial constraints arise from ethical challenges and a fear of the loss of emotional, interpersonal exchanges, especially in industries with significant human interaction, such as healthcare and education.
Vertical Solutions Always Expand Horizontally Across Their Domains
Vertical AI players inevitably evolve to integrate into broader ecosystems within their domains. This progression is driven by end user’s preferences to accomplish their tasks through one or two interfaces rather than switching between multiple specialized applications. Consequently, the long-term success of vertical solutions hinges on their ability to go horizontal within their specific domains, creating a flywheel effect that drives further integration and value creation.
To understand this transition, it helps to examine the rise of vertical software. Vertical software companies typically follow a similar playbook:
- Gaining adoption with a focused use case: They start with a specific, well-defined problem.
- Expand into adjacent capabilities: They expand by adding related features and functionalities that complement the initial use case.
Examples include Shopify (starting with e-commerce and expanding into payments and shipping as well as Pos) and Slack (beginning with internal team communications and expanding into project management tools and customer support software), or Zendesk (initially offering customer support ticketing and expanding into a full product suite including sales automation, chat support, and customer engagement tools). These companies successfully scaled by first unbundling a specific service to solve a single pain point, embedding themselves in customer workflows, and then re-bundled it with additional capabilities to provide a comprehensive solution.
The reason this strategy works is that while disruption often starts with unbundling established services, most venture returns are realized through the subsequent capabilities re-bundling process. By integrating and expanding their offerings, vertical AI players can capture greater value and build more sustainable, scalable businesses.
Thesis Map
Vertical AI/Agents Opportunities
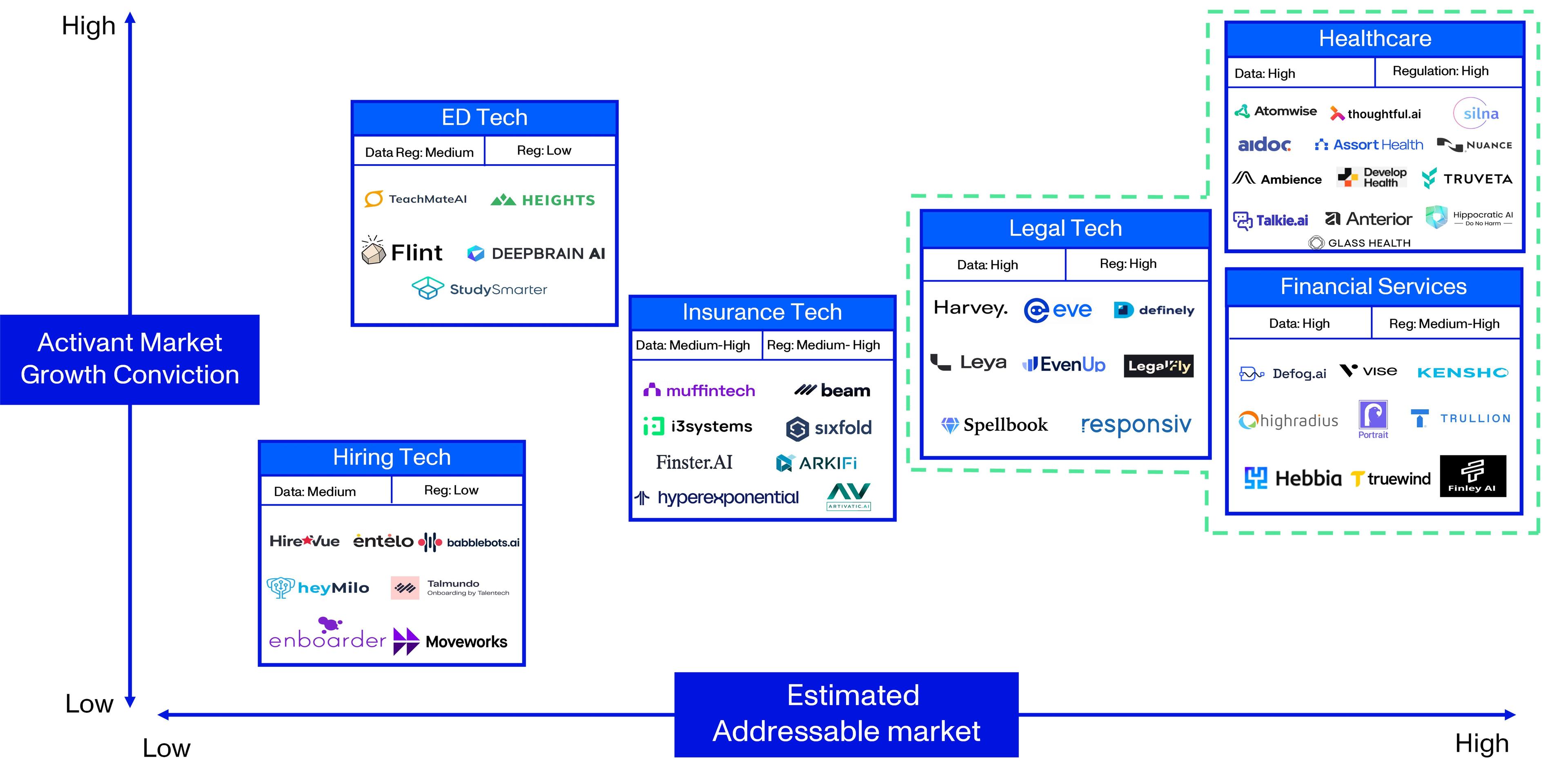
We believe as seen in the exhibit above that Healthcare, Financial Services, and Legal Tech represent the industries with the greatest potential for disruption by Vertical AI Agents. These sectors are characterized by massive market sizes, a wealth of available data, stringent regulatory requirements, and historically slow technology adoption. Additionally, they rely heavily on human input for repetitive, data-driven, and highly specialized tasks—creating a perfect storm for vertical AI solutions.
Conclusion
Organizations have long used offshoring, outsourcing, and contract work to access external human resources. However, the introduction of AI agents is changing this landscape, enabling technology to perform tasks previously managed by humans. Unlike external human resources, AI agents can easily be directly embedded into organizations.
This shift marks the beginning of a new phase in technology, where AI agents form structured networks that align with organizational objectives. These AI agent fleets will interact and require new communication protocols for efficient collaboration. We are witnessing AI agents becoming specialized in various verticals, adopting specific industry operations. The growing market of autonomous AI agents will lead to the creation of marketplaces, where hiring an AI agent will become as straightforward as hiring a freelancer on platforms like Upwork or Fiverr.
As AI agents become more widespread, compliance and liability issues will emerge as significant challenges and opportunities. Organizations that effectively manage these aspects will gain a competitive advantage, particularly as AI agents operate independently.
In our previous research on revenue operations (RevOps), we explored how the advanced capabilities of AI agents can pave the way towards Virtual AI Employees and highlighted the significance of cross-functional data alignment. Combined with the capabilities of vertical AI, the greatest value emerges when these players expand their reach horizontally within the vertical specific domain, gradually integrating additional business functions to form an AI agent workforce rather than relying solely on standalone point solution agents. Companies like 11x are taking significant steps in this direction, starting with a virtual outbound SDR and expanding into other areas such as RevOps and call centers. We believe that advanced vertical players, such as Harvey, Finley AI, and others who successfully extend their capabilities horizontally, will be the winners in this market.
Endnotes
[1] Thomson Reuters, Future of Professionals Report, 2024
[2] Verdict, VC funding: Can AI spur edtech back towards 2021 levels?, 2024
[3] Accenture, Research Report “A New Era of Generative AI for Everyone”, 2023.
The information contained herein is provided for informational purposes only and should not be construed as investment advice. The opinions, views, forecasts, performance, estimates, etc. expressed herein are subject to change without notice. Certain statements contained herein reflect the subjective views and opinions of Activant. Past performance is not indicative of future results. No representation is made that any investment will or is likely to achieve its objectives. All investments involve risk and may result in loss. This newsletter does not constitute an offer to sell or a solicitation of an offer to buy any security. Activant does not provide tax or legal advice and you are encouraged to seek the advice of a tax or legal professional regarding your individual circumstances.
This content may not under any circumstances be relied upon when making a decision to invest in any fund or investment, including those managed by Activant. Certain information contained in here has been obtained from third-party sources, including from portfolio companies of funds managed by Activant. While taken from sources believed to be reliable, Activant has not independently verified such information and makes no representations about the current or enduring accuracy of the information or its appropriateness for a given situation.
Activant does not solicit or make its services available to the public. The content provided herein may include information regarding past and/or present portfolio companies or investments managed by Activant, its affiliates and/or personnel. References to specific companies are for illustrative purposes only and do not necessarily reflect Activant investments. It should not be assumed that investments made in the future will have similar characteristics. Please see “full list of investments” at https://activantcapital.com/companies/ for a full list of investments. Any portfolio companies discussed herein should not be assumed to have been profitable. Certain information herein constitutes “forward-looking statements.” All forward-looking statements represent only the intent and belief of Activant as of the date such statements were made. None of Activant or any of its affiliates (i) assumes any responsibility for the accuracy and completeness of any forward-looking statements or (ii) undertakes any obligation to disseminate any updates or revisions to any forward-looking statement contained herein to reflect any change in their expectation with regard thereto or any change in events, conditions or circumstances on which any such statement is based. Due to various risks and uncertainties, actual events or results may differ materially from those reflected or contemplated in such forward-looking statements.