Topic
Automation
Published
December 2023
Reading time
19 minutes
Industrial Intelligence
Why manufacturing needs software now more than ever
Industrial Intelligence
Download ArticleResearch
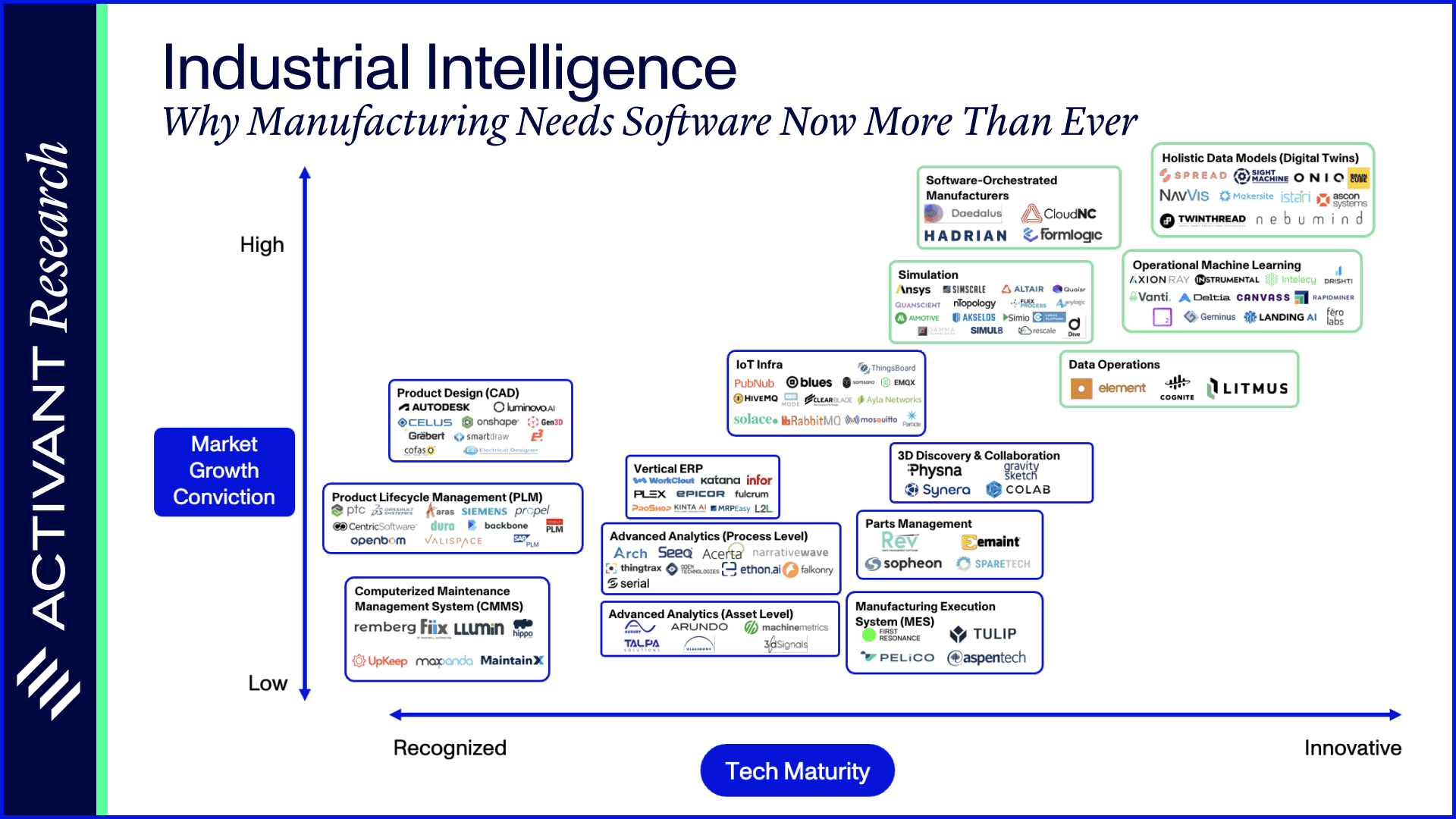
Industrial Innovation has stalled
In 1908, Ford unveiled the Model T, a four-cylinder, 20-horsepower car that cost $850. Production was painfully slow, with factories only able to make ~32,000 per year by 1910.1
However, Ford cracked the code on something no one else had solved: part interchangeability.2 This innovation, followed by the introduction of the assembly line in 1913, which broke tasks down into smaller, specialized jobs, cut the average task cycle time for a Ford assembler by an order of magnitude, from 514 to 1.9 minutes.
The result? Just a few years later, Ford had increased the output of Model Ts by a whopping 23x and cut its price by more than half - a tectonic shift that single-handedly launched the automotive age.3
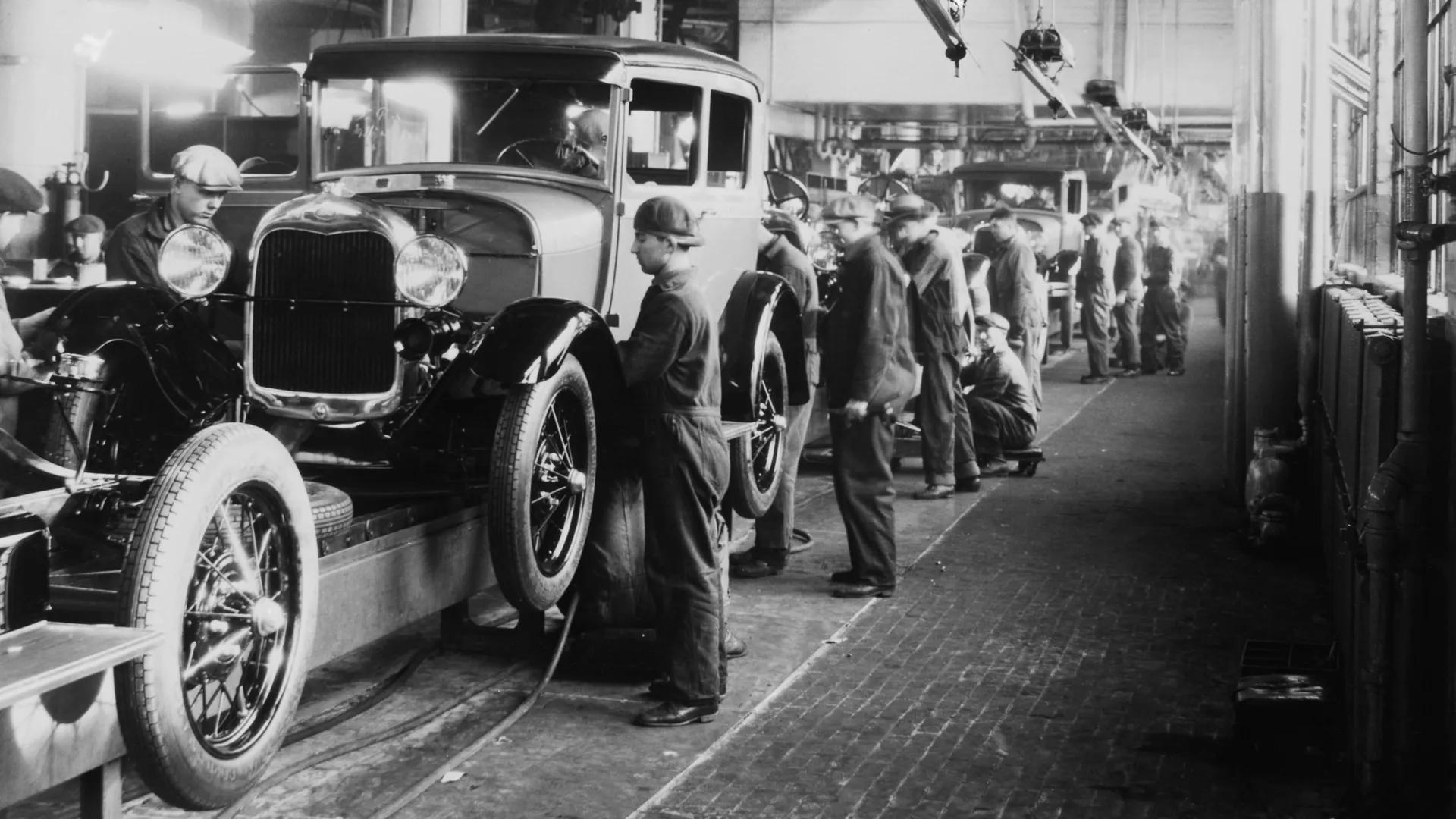
Ford's methods continued to define manufacturing, with future waves building on the system. Lean manufacturing, for example, honed in on waste and inefficiency and gained popularity in the 1950s via its success at Toyota.4 In the 80s came Six Sigma, which brought an obsessive focus on quality - with managers using statistical analysis to greatly reduce variation and defects.5
But recently, we’ve hit a wall. The progress Ford began over a hundred years ago has largely stalled: even if a factory has 21st-century machinery, it’s often still managed using 20th-century methods.
We believe that’s all about to change - and not a moment too soon. In this piece, we explore the transformative potential of industrial intelligence, and how it's (finally) poised to drive the next wave of efficiency and sustainability in manufacturing.
What is Industrial Intelligence
Industrial Intelligence is the sub-sector of Industrial software that leverages enterprise data to add an intelligence layer to industrial operations. It’s the application of new technologies, like modern data infrastructures, analytics, machine learning, and digital twins to the industrial sector. Industrial Intelligence means unlocking all the operational data that these organizations generate and leveraging it to drive efficiency, increase returns, and meet sustainability targets.
At this point, you must be asking yourself – how have the world’s leading manufacturers not innovated on their technology infrastructure already? Let’s dive in.
How we got here
Like many “old economy” sectors, the industrial space has largely been left behind by the past decade of software innovation. Here’s why:
- Buying cycles are on par with government procurement (read: glacial). Industrial firms often require lengthy proof of concept programs before making a buying decision. Even if ROI is proven, there’s still a bidding process, which further harms success rates. Only a few can stomach the wait - or the risk.
- Niche manufacturers have less incentive to innovate. If you’re the manufacturer of a particular spring used in a seatbelt retractor for a Porsche 911, odds are you have very little competition. Without competition, the status quo is fine.
- Complex manufacturing environments need complex implementations. Every customer has a unique combination of legacy infrastructure and future needs. Combine that with a byzantine and often incompatible manufacturing hardware landscape, and you quickly realize why adding a software layer is so difficult.
- “Never touch a running operation” is an oft-repeated mantra, and for good reason. On average, switching off just a single BMW factory for only one day could cost ~$12mn.6
- Cultural inertia remains a challenge. Telling a tenured production manager who “has been doing this for 30 years” that there might be a better way is never easy, especially when historic “buzzy” areas of manufacturing innovation failed to prove value until now, like 3D printing in the early 2010s.
Taken together, it's no surprise industrial software has come up short - with real costs to companies and consumers alike.
Big challenge, bigger opportunity
Legacy software lets its users down. Why, you ask? For starters, the companies developing it are not software-natives. Of the top 15 incumbents, only four are pure-play software vendors - (Dassault, Autodesk, PTC & Ansys). The rest (Siemens, etc.) are hardware players at their core, with software contributing less than 10% of their revenue.7 The quality of experience we’ve come to expect from consumer and now business software is hard to come by in the industrial sector:
Leaders find it to be more challenging than ever to engineer high-quality products, as their race to incorporate novel technologies introduces unforeseen challenges. There’s now more data about problems available to engineering teams than ever before. But because the existing manufacturing analytics toolkit is legacy, teams often spend their day wading through messy data, instead of solving problems.”
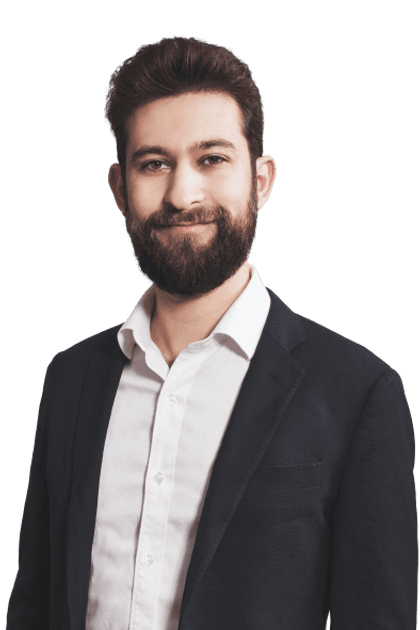
Daniel First, Founder & CEO, Axion Ray
Nevertheless, many incumbents realize the value of diversifying to recurring, high-margin software earnings in what is historically a cyclical sector - and they have the pockets to go shopping. Rather than develop products organically, many larger players are building out vertical-specific software offerings via acquisitions. Since 2021, for example, Siemens spent ~$2b on buying predictive maintenance tools, while Emerson and Schneider acquired industrial software giants AspenTech and Aveva for ~$11b and ~$10b, respectively.8 This inorganic approach of legacy vendors creates two major pain points in the industry:
- Compartmentalized, ‘Frankenstein’ stacks. For instance, Rockwell’s Design software suite consists of seven different sub-products, among which two were acquired (Emulate3D & Arena) and one accessed via partnership (FactoryTalk Twin Studio).
- Complex offerings make plug-and-play extremely difficult. Instead, they require significant customization to roll out. Incumbents advertise this “flexibility” as a “feature”, but most operators hate the need to call their service provider for every small change (and the ensuing bill).
Another challenge is that industrial software needs to solve industrial-scale issues. For one, analyze immense volumes of data: manufacturing plants generate twice as much data as any other vertical.9 Per week, a typical smart factory generates ~5PB (that’s petabytes, or one million gigabytes).10
Throw that at your average legacy software, and more issues quickly come into focus:
- Complex infrastructure is fertile ground for data siloes. Across the board, experts mention that data often remains stuck on the production-line level. This leads to incomplete (meta-) data, duplicate processes, and barriers to collaboration. Most importantly, however, it hides operational insights - or in other words, better ways to run the factory. In a survey of 1,022 respondents, 68% reported that data siloes have a negative impact on their work. Those workers were found to spend 11.6 hours per week searching for the information they needed.11
- Engineers lack the tools to move right into analysis. Data engineers still spend around 40% of their time on data quality – time spent not solving problems.12 We routinely hear of engineers still exporting data from faulty machines to Excel, only to spend days wrestling with it.
- Once you get to the analysis, doing it manually is grossly insufficient. Manufacturing processes generate immense volumes of data. Finding issues can be like searching for a needle in a haystack – that’s why human analysis will always be too late to be truly impactful.
Modern hardware – electric vehicles for instance – have extremely complex software. Manufacturers need to shift from an annual product cycle to daily software updates, but engineers are stuck solving problems in Excel. The complexity inherent in manufacturing modern products is rising exponentially, and organizations need the software they use to step up accordingly.”
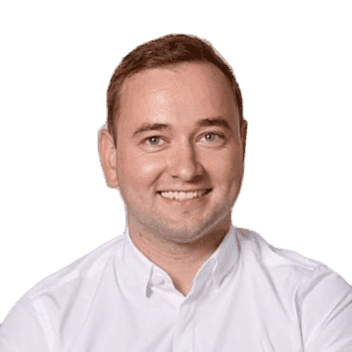
Robert Göbel, Co-founder & Managing Director, Spread.AI
In short, there is a massive opportunity to challenge incumbents’ complexity and unlock the insights hidden in operational data - by offering software that is quicker to deploy, easier to customize, cloud-native, and collaborative.
With improved intelligence tools, manufacturers can reduce downtime, improve quality, and increase throughput – aka increase profitability. And crucially, the technical underpinnings for this shift have finally fallen into place.
A revolution in the making
In the past decade, we have seen three key technologies reach maturity: namely, our ability to collect, store, and analyze data on an industrial scale.
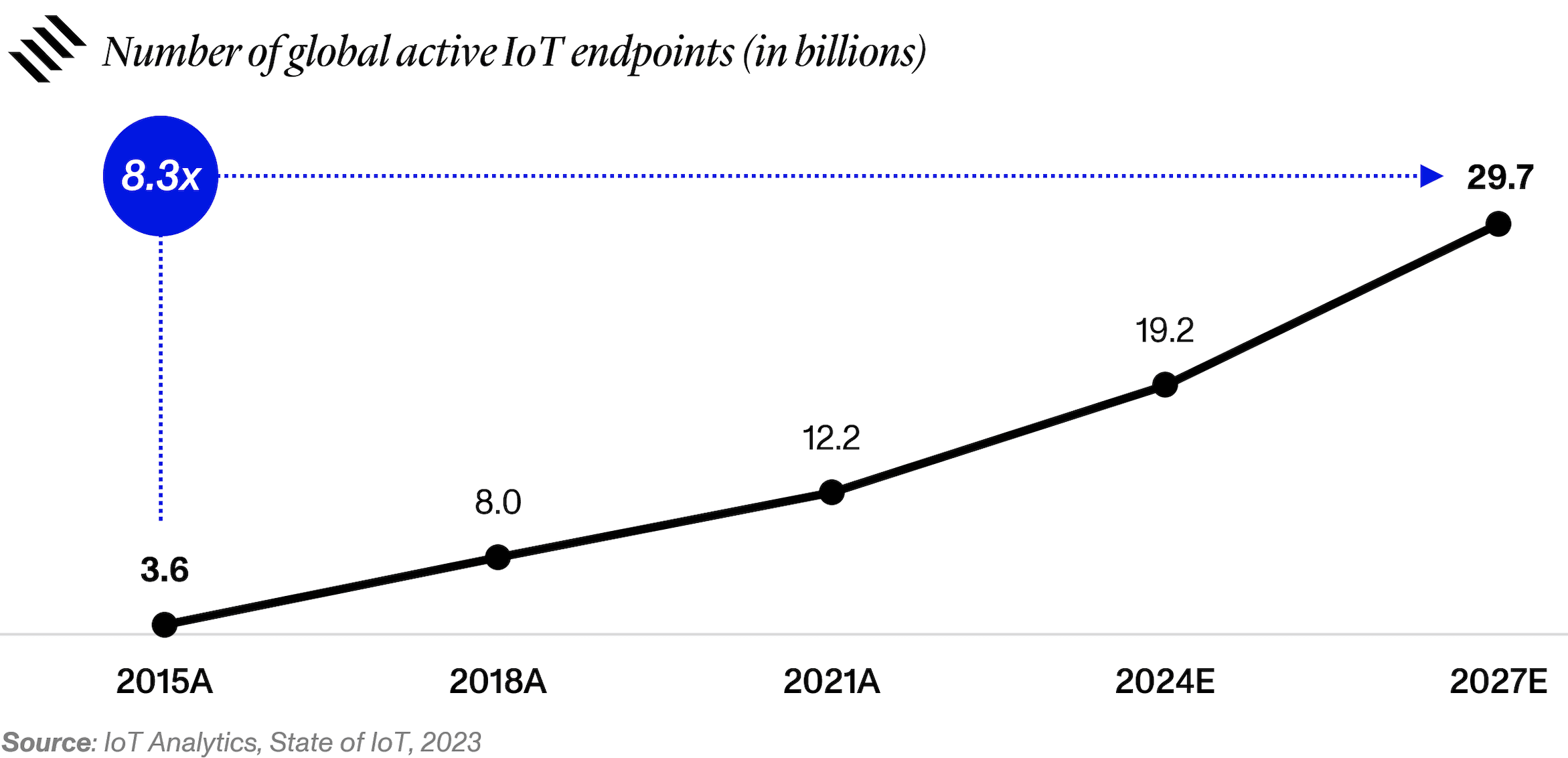
Let’s start with data collection. In 2004, an average IoT sensor cost $1.30. By 2020, the cost had fallen by almost 4x to $0.38.13 IoT has benefitted from the relentless innovation in semiconductor technology & connectivity protocols, driving down costs and improving performance. We don’t expect this trend to slow anytime soon - if anything, just the opposite. As IoT sensors multiply, so too will the volume of operational data available to manufacturers. Companies like HiveMQ are supporting the infrastructure of this significant tailwind with their MQTT platform, which allows customers to send and analyze data from IoT devices in real-time and even manipulate data in motion to ensure integrity and quality across deployments.
Or consider storage. Just years ago, these new flows of data would’ve overwhelmed the average data center. But since 2010, the cost of cloud storage has roughly halved, and the number of industrial firms who have completed a shift to the cloud has risen from 13% to 64%.14,15,16Now, it is finally feasible (and cost-effective) for manufacturers to store this data for later use.
Meanwhile, improvements in database technology open the door for effective analysis. Time-series databases like Druid and TimescaleDB are key to handling the mass of time-series data produced by industrial firms, especially process manufacturers. Moreover, as industries increasingly embrace electrification and software-driven "smart" products, graph databases have become instrumental in understanding the interrelationships between mechanics, electronics, and software.
Finally, data analysis has also benefited from rapid advances in processing technology like the data warehouse, which has greatly reduced both latency and cost. A prominent example is Snowflake, which has recently introduced a vertically designed manufacturing cloud to the market.
Taken together, these technologies finally make possible a “single pane of glass” through which industrial organizations can monitor and analyze their operations in real-time. This is the value of true industrial intelligence – it makes data accessible, paving the way for value creation via analytics, machine learning, and digital twins.
The need has never been greater
Many industrials are facing a steep knowledge cliff: 75% of machine operators in the US are over the age of 45, yet the average 18-year-old does not consider manufacturing as their first-choice career.17 As staff retire and companies lose critical expertise, intelligent software will have to step in. The burden of running a factory must shift from accumulated experience to mathematical models.
And in a Western world short on skills, the demand for reshoring just adds fuel to the fire. In the US, reshoring-linked jobs are skyrocketing – up 6.5x from 2012 to 2022.18Add geopolitical tensions, the lingering effects of Covid-19 or the Inflation Reduction Act, and you see why solutions are so desperately needed. Estimates are that organizations will need to spend ~$60b to rebuild supply chains in the coming years on software alone.19
Critically, software can also play a key role in our journey to net-zero. Until recently, for example, Unilever’s Indaiatuba plant in Brazil (which makes powder detergent) was their largest greenhouse gas emitter. By applying machine learning to the spray-drying process, however, the company was able to reduce GHG emissions by 96%.20
In fact, the average company applying these technologies sees improvements not just in energy use (-24%), but also in productivity (+52%), labor costs (-32%), and manufacturing costs (-26%).21 The scale of the opportunity, both ecologically and economically, is massive. In our analysis, we assume that the global manufacturing sector could save ~25% of its COGS, in line with the examples seen above. The resulting resource savings would be equal to the entire GDP of France.
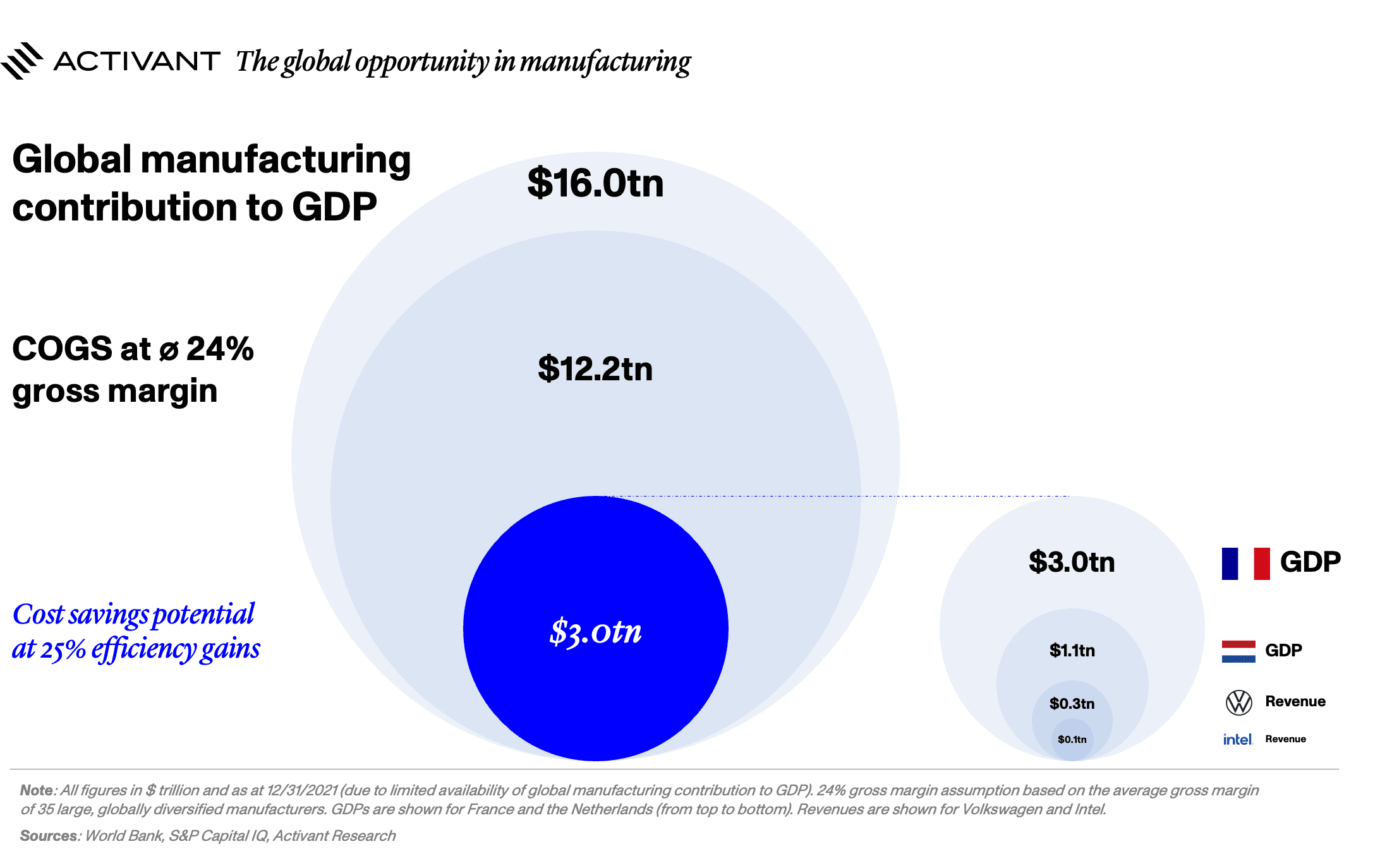
Moreover, savings are only one side of the equation. Efficiency gains are a huge resource unlock, and it's impossible to put a number on the upside potential inherent in giving engineers more time to focus on building great products. Our estimate: It will be big, given the sheer size of this industry.
Thesis Map
Our View on Industrial Software
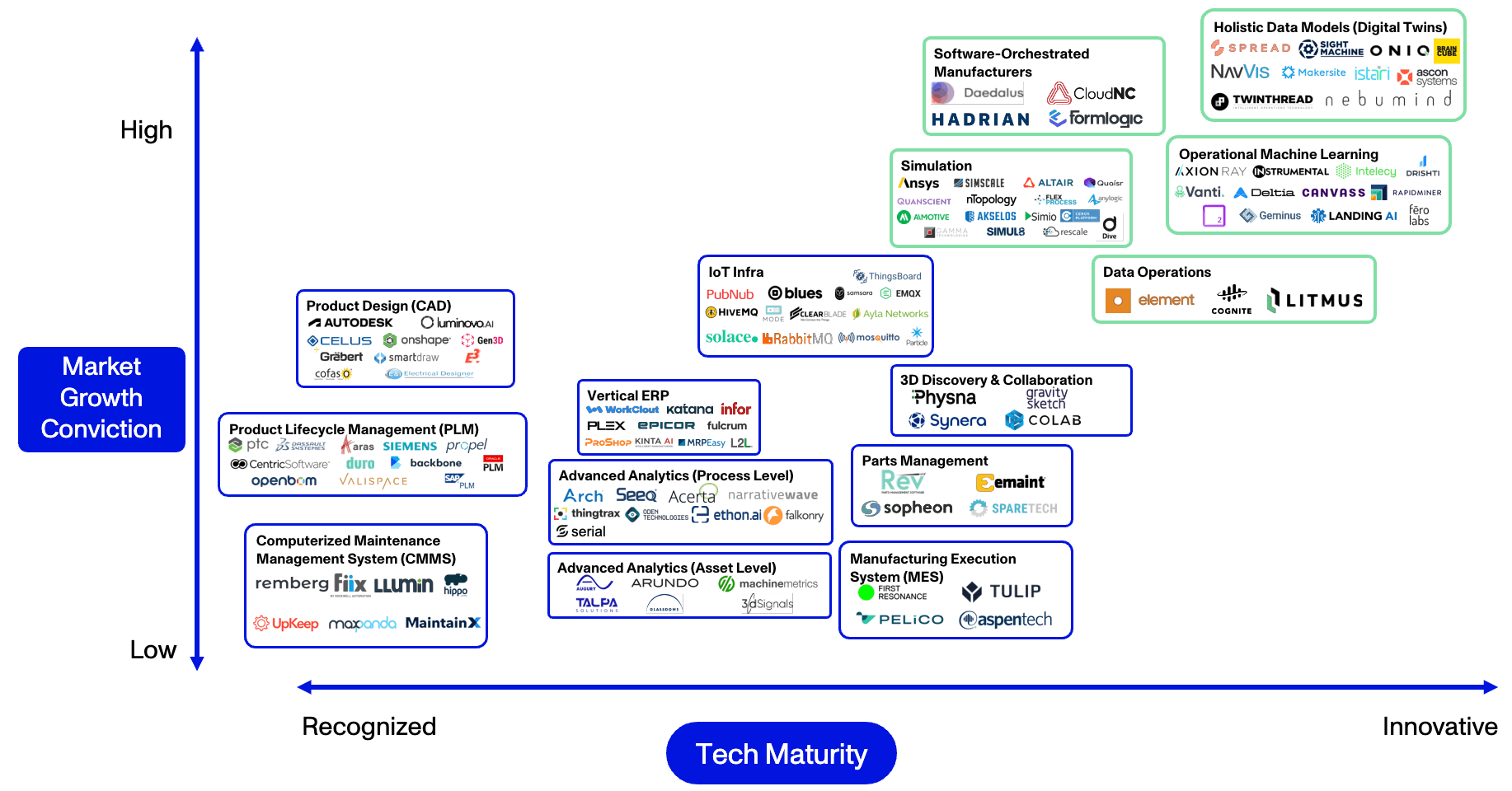
So, when we look across the whole Industrial Software landscape, which companies do we see as the most innovative and most likely to see durable growth through the next decade? It is those companies that are working to add the intelligence layer to industrial operations: Data Operations, Operational Machine Learning and Digital Twins. We’ll highlight a few of these, together with one segment that is mature but showing bright spots of innovation – Simulation.
1Holistic Data Models (Digital Twins)
In our view, Digital Twins are the most innovative segment of this exciting market. They combine (some or all of) 3D rendering, physical simulations, and real-time operational data to provide a digital representation of physical products and their assembly lines. In other words, they are considered a “holy grail” in industrial intelligence, as they bring together 1) a data platform, 2) analytics and machine learning, and 3) automation and operationalization capabilities. As such, this segment is growing rapidly and is expected to reach $46b by 2027, up from $13b today (a 36% CAGR).22 A notable player here is Spread.ai, whose graph database-based engineering intelligence software provides a holistic view of manufactured products to power use cases across the engineering lifecycle. For example, Spread supports auto OEMs on the shift toward EVs with an understanding of how mechanics, electronics, and software interact.
As this segment matures, agile start-ups will face incumbents like Siemens & PTC, whose advantages include owning existing hardware, extensive consulting teams, and recognizable brands. However, most of the users that we’ve spoken to have questioned whether these incumbent products meet the mark. We think that the door is now open to challengers who can build exceptional technology.
2Operational Machine Learning
Today, companies either provide pre-trained models to perform specific analyses, or no-code / intuitive interfaces that allow process engineers and other operational personnel to train and deploy machine learning models for specific needs. Players in the sector tend to specialize: Canvass primarily serves oil refineries, while Basetwo targets drug and pharma vendors, for example.
The application of ML/AI to highly specialized use cases can drive significant ROI for customers, as we’ve seen in the case of Unilever’s Indaiatuba plant. As such, these companies can see a quick time to value and become deeply integrated in their customer’s workflows. Competition is typically lower as vendors focus on their respective verticals. Market opportunity is a key consideration, however: we see the total Industrial MLOps & ML-as-a-Service market reaching $28.7b by 2028.25 Niche vendors may need to make the leap to other industries to allow for breakout potential or cut across industrial verticals like Axionray or Instrumental. Where Axionray leverages natural language processing, Instrumental leans heavily on image data. Both are tapping masses of unstructured data to detect quality issues long before a human could and they’ve seen strong adoption from large enterprises like PEM, Boeing, Meta & Cisco – a testament to the value they can create.
3Engineering Simulation
Simulations help engineers close the gap between design and reality and see how their designs will - or won’t - behave in millions of real-world scenarios. This can reduce or even eliminate the need for costly physical testing. Simulation is a mature market, but there is innovation occurring at the edges. Cloud-native simulation is making it more accessible and cost-effective to run simulations, eliminating the need for expensive on-premises workstations. Leading the charge in this innovation are Simscale, based in Munich, and Dive, located in Berlin. Dive is particularly noteworthy for its pioneering particle-based simulation, opening entirely new use cases – like understanding washing systems, liquid cooling, and drivetrain lubrication.
4Data Operations
For too many industrial companies, data is still largely inaccessible and disconnected. In fact, most companies spend more time finding and manipulating data, rather than extracting any value from it. Data Ops solves for this by systematically and effectively managing data so that it can be contextualized and used for decision-making - fast. In parallel with IoT, the market is taking off and is projected to reach $11b by 2028, growing at a 23% CAGR.31 One notable player: Cognite, which offers a data operations and contextualization platform designed to power digital twins for industrial applications.
5Software-orchestrated manufacturers
To see the full benefits of Industrial Intelligence realized, some companies are making a strategic shift. By moving away from selling software and towards building modern factories from the ground up, these companies are no longer constrained by legacy and fragmented technology – they can start afresh using a software-centric blueprint. This can massively expand the TAM too – Industrial Software is a large market, but global manufacturing is a $16 trillion market!
Daedalus, from Germany, uses their proprietary FactoryOS to orchestrate a connected factory and become the central data layer – driving constant improvement and automation to increase the quality & throughput of the parts they produce. Both Hadrian and Formlogic follow a similar approach and are also using their proprietary software to orchestrate and automate precision manufacturing operations, hopefully becoming the backbone of this industry in the US.
The North Star: Driving Return on Investment (ROI)
Industrial Intelligence start-ups hold significant potential to deliver substantial value to their customers. Nevertheless, breaking into the industrial market remains a formidable challenge. To succeed, companies must not only build complex, high-quality technology but also pair that with a robust go-to-market approach. Recall that many of the challenges in this market are structural, and if technology is not the problem, then only building more technology will not necessarily solve it.
Companies should make generating ROI their north star - but when it comes to the Industrial sector, “we’ll make your business more efficient” just won’t cut it. In our view, the most successful challengers are laser-focused on defining a very specific use case for their product. This provides the basis to demonstrate tangible, quantifiable ROI. Finally, we see the best companies aligning their go-to-market approach and technology roadmap with these targeted use cases, making rapid ROI the flywheel that drives the whole business.
Case Study
Driving ROI
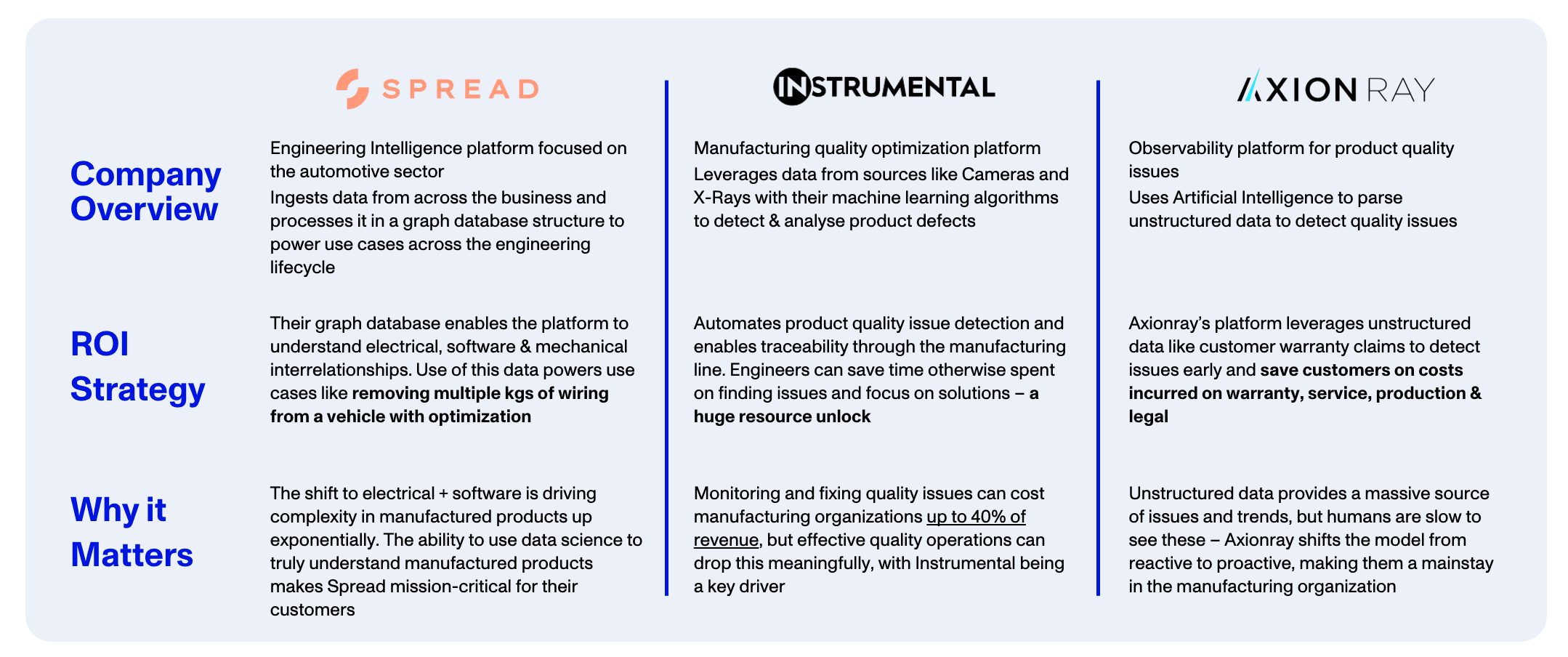
Accelerating an industry
The industrial sector is the backbone of our modern society. The proliferation of IoT sensors, the ever-decreasing cost of storage, and the introduction of vertical-specific AI use cases serve as the catalyst for this massive technical shift. This transformation is occurring just as society is so deeply in need of software-driven solutions, wrestling with skills gaps, reshoring needs, and climate goals.
At Activant, we think the “why now” case for this market has never been stronger. Despite their immense potential, the penetration of these modern technologies remains low. There is enormous upside to be captured. That’s why we’re fired up by the incredible founders we have met who are building the next era of software to level up industrial firms and transform this $16 trillion industry.
If you’re building in this market, please get in touch!
Footnotes
[1] Public Broadcasting Service, They Made America, 2004
[2] The Machine that Changed the World, The Story of Lean Production – Toyota’s Secret Weapon in the Global Car Wars That is Revolutionizing World Industry, 1990
[3] Public Broadcasting Service, They Made America, 2004
[4] Marshall Advanced Manufacturing Center, Lean Manufacturing Made Toyota the Success Story it is Today, 2007
[5] Project Management Institute,Six Sigma Method and Its Applications in Project Management, 2002
[6] BMW, BMW Group Report 2022, 2023, Activant Analysis
[7] Company Filings, Activant Analysis
[8] S&P CapitallQ, Activant Analysis
[9] International Society of Automation, What’s next for big data in process manufacturing, 2018
[10] Techerati, How data will build the factories of the future, 2018
[11] Forrester, The Crisis of Fractured Organizations, 2022
[12] Wakefield Research, State of Data Quality Report, 2023
[13] Wipfli LLP, Six factors that determine IoT pricing manufacturers, 2021
[14] AWS, S3 pricing, 2023 ; AWS, S3 pricing, 2010 (Wayback Machine)
[15] IBM, The power of cloud, 2012
[16] McKinsey, Clearing the air on cloud: How industrial companies can capture cloud technology’s full business value, 2021
[17] Deloitte, Creating pathways for tomorrow’s workforce today, 2021
[18] Reshoring Initiative, 2022 Data Report, 2023
[19] Bank of America, Digital Machination V: Payback Time, 2022
[20] World Economic Forum, Global Lighthouse Network: Shaping the Next Chapter of the Fourth Industrial Revolution, 2023
[21] Bank of America, Digital Machination V: Payback Time, 2022
[22] JP Morgan, A Soft Spot in a Potentially Hard Landing: Launching Coverage on Industrial Software, 2022
[23] Bank of America, Digital Machinations V: Payback time, 2022
[24] Research and Markets, Global Industrial Software Market Report, 2022
[25] KBV Research, Machine Learning Operationalization Management Market Size, 2022
[26] Allied Market Research, Machine Learning Operationalization Management Market Size, 2022
[27] World Bank, Manufacturing value added (% of GDP), 2023
[28] Precedence Research, Machine Learning as a Service Market Size, 2023
[29] Allied Market Research, Machine Learning as a Service Market Size, 2022
[30] World Bank, Manufacturing value added (% of GDP), 2023
[31] Markets and Markets, DataOps Platform Market, 2023
Disclaimer: The information contained herein is provided for informational purposes only and should not be construed as investment advice. The opinions, views, forecasts, performance, estimates, etc. expressed herein are subject to change without notice. Certain statements contained herein reflect the subjective views and opinions of Activant. Past performance is not indicative of future results. No representation is made that any investment will or is likely to achieve its objectives. All investments involve risk and may result in loss. This newsletter does not constitute an offer to sell or a solicitation of an offer to buy any security. Activant does not provide tax or legal advice and you are encouraged to seek the advice of a tax or legal professional regarding your individual circumstances.
This content may not under any circumstances be relied upon when making a decision to invest in any fund or investment, including those managed by Activant. Certain information contained in here has been obtained from third-party sources, including from portfolio companies of funds managed by Activant. While taken from sources believed to be reliable, Activant has not independently verified such information and makes no representations about the current or enduring accuracy of the information or its appropriateness for a given situation.
Activant does not solicit or make its services available to the public. The content provided herein may include information regarding past and/or present portfolio companies or investments managed by Activant, its affiliates and/or personnel. References to specific companies are for illustrative purposes only and do not necessarily reflect Activant investments. It should not be assumed that investments made in the future will have similar characteristics. Please see “full list of investments” at https://activantcapital.com/companies/ for a full list of investments. Any portfolio companies discussed herein should not be assumed to have been profitable. Certain information herein constitutes “forward-looking statements.” All forward-looking statements represent only the intent and belief of Activant as of the date such statements were made. None of Activant or any of its affiliates (i) assumes any responsibility for the accuracy and completeness of any forward-looking statements or (ii) undertakes any obligation to disseminate any updates or revisions to any forward-looking statement contained herein to reflect any change in their expectation with regard thereto or any change in events, conditions or circumstances on which any such statement is based. Due to various risks and uncertainties, actual events or results may differ materially from those reflected or contemplated in such forward-looking statements.